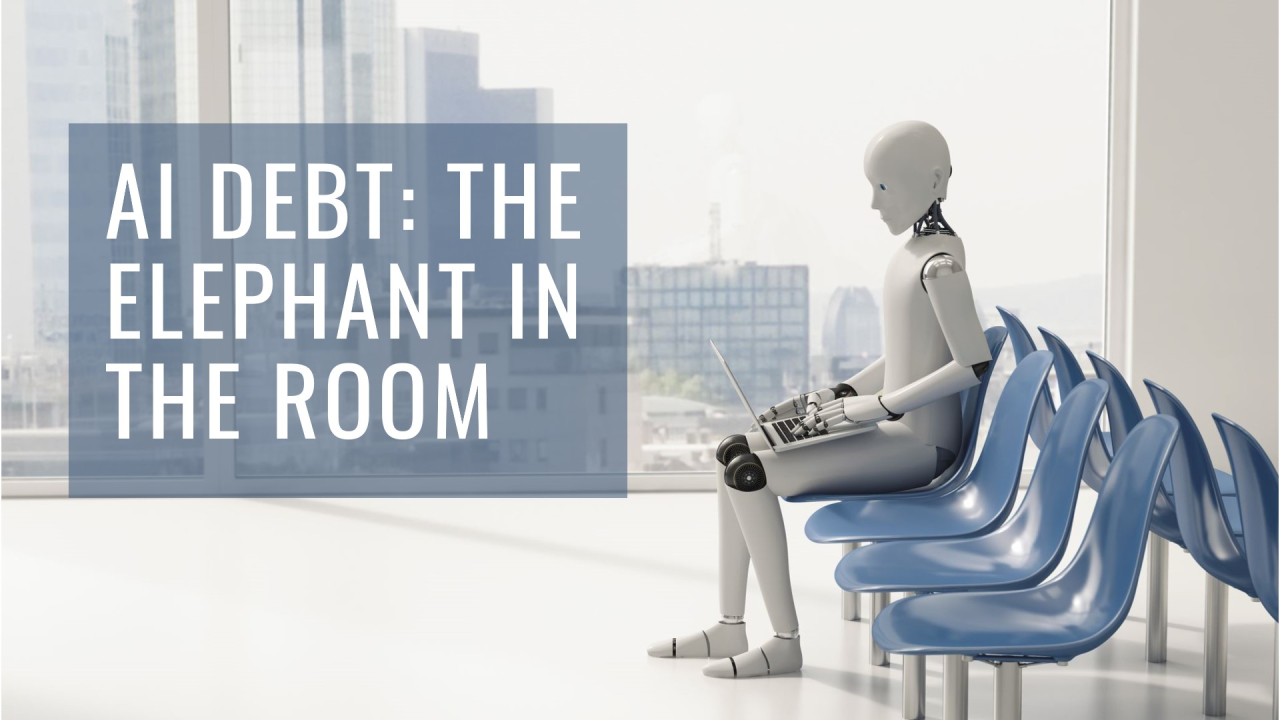
Introduction
I had a conversation with Christopher Bergh on my podcast last week The Data Strategy Show and this post is a consequence of our conversation. We discussed technical and data debt. Which made me think will organisations open themselves up to large amounts of AI Debt! The answer is I guess they will. My article today, explores this theme and will go some way to tackle some of the issues and offer some light relief for your budget.
We know that many organisations today are rushing to implement AI, eagerly purchasing tools and platforms to solve their so-called challenges. I think we can guess that there is a large amount of hypnosis occurring with many organisations and the usual hype cycle of shiny new toy syndrome is now making its presence known with AI.
But here’s the problem, I think many organisations today aren’t buying said objects with a considered long-term strategy in place, now they are actually buying with a short sightedness that will get them into even more trouble when the competition comes a knocking!
Why do I believe this? Because in my experience and what I’ve seen in many industries is that most are neglecting to design AI around their business needs, starting with use cases, and ensuring that data is prepared to support AI effectively.
This short-term thinking is leading organisations down a path toward what we can now happily call AI debt.
So, what is AI Debt?
AI debt is much like technical and data debt, is the accumulation of quick fixes, poorly integrated systems, and rushed implementations that are typically botched, and become costly to maintain, while hindering the future progress of the organisation. This AI debt is typically aroused when organisations adopt so-called AI solutions without a long-term vision, leaving their systems fragmented, their data in disarray, and their business strategies misaligned.
We saw it with Big Data, we are seeing it now, many are focusing on the shiny tools and platforms that promise instant results, you know the one where the vendor says “of course it can do that, and that, and that”! These organisations are falling into the same trap that they did with all the shiny toys that came before and again heralding the famous phrase “we will future proof” our organisation! I mean what codswallop! Many of these organisations will live with the age-old problems of platforms and tools, with no ability to scale, or worse, they won’t evolve with the organisation’s needs, and will be summarily dismissed in someone’s top drawer never to be seen again!
Frothing at the Mouth: Tools First, Use Cases Later
Okay so we know that the hype cycle works, we have to accept that people will always fall for it and in this particular cycle many are rushing to implement AI with the misguided belief that it will solve all their problems that have been swept under the carpet, by the generations before!
But come on, you and I know that AI is not magic right? We do, don’t we? AI is just a tool that requires well-structured use cases and clean data to deliver results. How dare you say it’s just a tool!
As per usual too many businesses are putting the cart before the horse, building AI capabilities without first identifying their specific business needs and addressing underlying data issues.
The result? AI models that are only as good as the data they consume, leading to poor outputs, biased predictions, and costly rework.
The design of AI into your organisation must lead with business use cases, not tools. You should assume AI is a consumer in this architecture, not the driver.
So, How Do We Future-Proof? <giggle>
My belief is that organisations need to radically rethink their approach to AI. Instead of seeing AI as an end in itself, it must be part of a broader, use case-driven strategy that prioritises value creation. This shift in mindset requires:
- Use Case-Centric Design: AI should not be implemented just because it’s the hot trend. Every AI initiative must be grounded in a clear business use case that solves a specific problem or meets an identified opportunity. Use cases guide the design of the necessary data structures, ensuring that AI is a tool for solving targeted business challenges.
- Laying the Data Foundations: After identifying your business use cases, ensure that your data architecture is designed to support them. Can you imagine that it might mean you will need some data architects. Oh, suits you sir! AI should be treated as a consumer of high-quality data that has been cleaned, integrated, and governed. Without this foundation, AI implementations will fail to deliver real value.
- Flexibility for the Future: I remember when I did my degree we learned about systems design, reading Codd and others, long time ago! With that said, systems must be designed to accommodate future advances in AI and data science. The tools of today won’t necessarily meet the demands of tomorrow. Choose technologies and platforms that can evolve, or better yet, focus on developing a data and AI operating model that ensures a level of flexibility and scalability. Designing for long-term value will help protect the business from becoming locked into rigid systems that can’t deliver sustainable growth.
- Cross-Functional Collaboration: AI doesn’t exist in a silo. Its success depends on collaboration across the business. AI strategies must be aligned with business objectives and involve cross-functional teams, including data engineers, business analysts, ethicists, and decision-makers. AI should be built into the fabric of the culture and how decisions are made across the enterprise, not tacked on afterward. This approach ensures that AI investments are driving value through every corner of the business.
- Governance and Accountability: As AI becomes more embedded into operations, accountability around its use is crucial. If we want to avoid AI debt it requires clear governance frameworks that dictate how data is managed, how models are maintained, and who is accountable for AI outcomes. AI governance is an extension which is required for societal, political, environmental, and human reasons! Again, this governance structure ties AI results directly to business impact, ensuring that every AI project generates measurable business value.
Value-Driven AI Is This The Way to Avoiding AI Debt
When I and my colleagues work with organisations to support their data and AI strategy, we avoid treating AI as a novelty or a technology experiment. Every AI initiative we uncover, must have a direct line of sight to value creation, and as mentioned earlier in this article, we need to begin with business use cases. Use cases provide the context and clarity for where AI can drive the most value. Once you identify the business challenge or opportunity, you can then design your data and AI strategy around that need.
This approach ensures that AI is applied where it will make the biggest impact, aligned with your business goals, and capable of delivering a clear ROI. Without this focus, AI initiatives will become disconnected from the core business, potentially wasting resources and delivering little to no value.
Here’s how to avoid falling into the AI death I mean debt trap and ensure you stay focused on use cases and value creation:
- Lead with Use Cases: Start with the business challenges or opportunities where AI can deliver real value. Whether it’s improving operational efficiency, enhancing customer experiences, or generating new revenue streams, AI investments must be tied to solving specific business problems. Once the use cases are clear, you can then design the data architecture that will support these AI solutions. This ensures that AI is focused on generating value rather than being implemented for its own sake.
- Design the Data Layer: Once your use cases are defined, focus on ensuring your data is clean, integrated, and governed in a way that supports the AI initiatives. The data should be tailored to the specific needs of the use case, ensuring that AI models are fed with accurate, relevant information that leads to valuable outcomes.
- Plan for Scale: AI solutions need to scale with your business, and so should your data infrastructure. Don’t just buy tools for today’s needs; invest in systems that can grow with your ambitions. Scalable systems ensure your AI investments continue to generate value as your business evolves, reducing your AI debt.
- Invest in Continuous Learning: AI models degrade over time if not maintained. Invest in teams and processes that can continuously improve and recalibrate AI models as your business needs evolve. This ongoing investment ensures that AI continues to generate value long after its initial deployment.
By grounding every AI initiative in a business use case and building the right data foundation around it, you ensure that your AI efforts are always aligned with value creation and drive sustainable business outcomes.
Avoiding the AI Trap
The AI landscape is hurtling forward, and I’m still baffled by how many organisations keep getting it wrong. They’re blindly chasing shiny new tools, burning cash on systems that won’t scale, stuck with AI that doesn’t deliver and worst of all, their data is a complete mess.
It’s shocking, really. How many times do we need to say this before it sinks in?
Listen, if you’re not thinking long-term, you’re heading straight into AI debt. Your systems will collapse under the weight of their own inefficiency, your tools will become outdated faster than you can blink, and fragmented data will choke any chance of real success. This is the brutal reality, yet so many organizations keep stumbling down this path.
So let me be blunt we MUST lead with business use cases. Not technical use cases, not data use cases but ones that are business focused. AI should solve real business problems, not just be a fancy tech toy. What about your data? It must be designed to support those use cases, or you’re just setting yourself up to fail. Also, please stop talking about how we will “future-proof” blah blah blah, implement AI systems that can scale, adapt, and evolve. No more half-baked solutions that crumble at the first sign of growth.
When you look back at your data and AI strategy the bottom-line value creation is the only thing that matters. If your data and AI strategy isn’t super focused on driving measurable business outcomes, you’re just pouring money down the drain. AI will only deliver on its promises when it’s built on a foundation of business value otherwise, you’re simply sinking into the debt cycle.
Stop repeating the same mistakes. Get it right this time or watch your AI dreams implode.